Surface Classification with CNN and LSTM Networks Based on Bicycle Vibration DataBachelor Thesis
20 July 2023, by Lars Melby
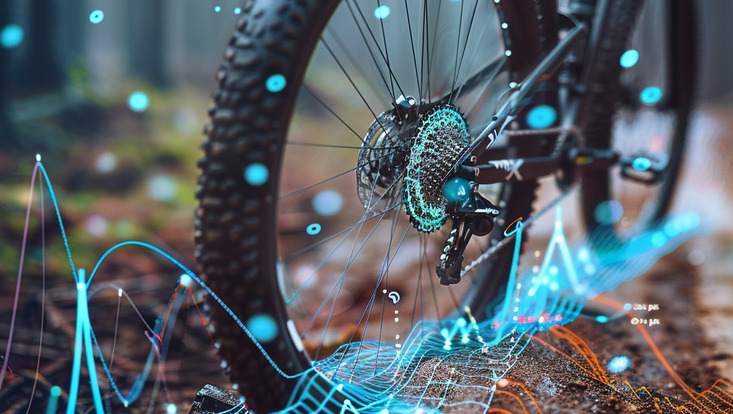
Photo: midjourney
Abstract:
This thesis focuses on promoting cycling as a sustainable transportation option to reduce carbon dioxide emissions. In Germany, the seamless expansion of the bicycle network plays a key role in promoting cycling. The objective of this thesis is to propose a vibration-based surface classification method for monitoring and identifying deficiencies in the bicycle network using Convolutional Neural Networks (CNNs) and Long shortterm memory Networks (LSTMs). A novel dataset was created, capturing vibration and velocity time series data during cycling, using a newly developed sensor box with an Arduino device and a dedicated mobile app for manual labeling. Extensive preprocessing techniques, including velocity normalization for the vibration data, were implemented to enhance dataset quality. Both CNNs and LSTMs exhibited remarkable accuracy in classifying surface types, with the CNN showing superior training stability and adaptability. These findings hold practical implications for effectively monitoring and maintaining bicycle infrastructure for seamless expansion.
Supervised by:
Dr. Wolf Posdorfer, Prof Dr. Janick Edinger