Machine-Learning enhanced densification for location-based sensor dataBachelor Thesis
27 March 2024, by Roman Tsymbal
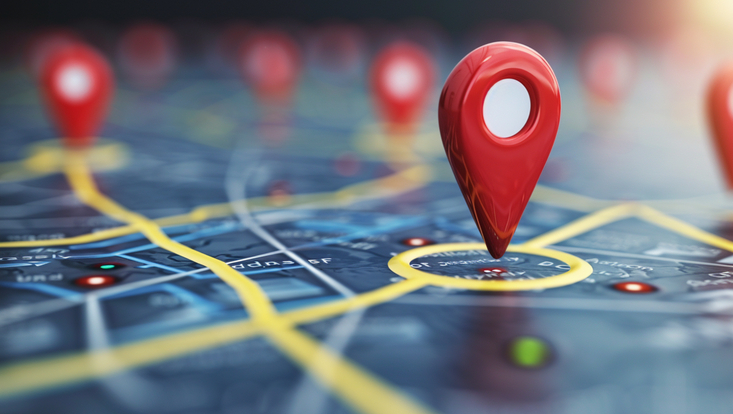
Photo: midjourney
This thesis examines the potential of machine learning techniques, particularly histogram-based gradient boosting, to enhance the interpolation of air temperature data in urban contexts, where the urban heat island effect poses a significant challenge. Traditional methods of temperature measurement, such as satellite data and ground-based weather stations, often suffer from low spatial resolution or gaps in data coverage, especially in densely built environments. To overcome these limitations, this study integrates machine learning with data from various weather sensor networks, including crowd-sourced networks like Netatmo and Sensor.Community.
A key focus of the research is enhancing the spatial and temporal resolution of air temperature data using mobile sensors deployed on buses and scooters as case studies. Through rigorous quality control measures, such as the CrowdQC+ framework, the collected sensor data is cleaned and prepared for accurate interpolation. The thesis demonstrates that machine learning models, by recognizing complex patterns in environmental data, can outperform traditional interpolation methods, providing more precise and reliable temperature predictions. In dynamic urban environments, where continuous, high-resolution temperature monitoring is of paramount importance for addressing the challenges associated with the urban heat island effect, these improvements offer significant benefits.
Supervised by:
Prof. Dr. Janick Edinger, Philipp Kisters