Prof. Dr. Sören Laue
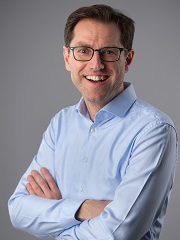
Professor for Machine Learning
Address
Office
Contact
Short Bio
Sören studied mathematics, physics, and computer science at the University of Leipzig and the University of Saarbrücken. He earned his Ph.D. in algorithmics and optimization from the Max Planck Institute for Informatics. After being a researcher for machine learning at the Friedrich Schiller University Jena, he became a full professor at the Technical University of Kaiserslautern, where he led the Algorithms for Machine Learning group. Since April 2023, he has been a professor at the University of Hamburg, where he heads the Machine Learning group. His distinguished research focuses on developing efficient algorithms and optimization methods for machine learning problems. He created the web service MatrixCalculus.org, which is used by more than 100,000 users annually.
Research
See the research pages of our group.
Publications
Selected Publications. A full list can be found here.
- M. Blacher, Ch. Staudt, J. Klaus, M. Wenig, N. Merk, A. Breuer, M. Engel, S. Laue, J. Giesen.
Einsum Benchmark: Enabling the Development of Next-Generation Tensor Execution Engines, NeurIPS 2024. - M. Blacher, J. Giesen, J. Klaus, Ch. Staudt, S. Laue, V. Leis.
Efficient and Portable Einstein Summation in SQL, SIGMOD 2023. - M. Mitterreiter, M. Koch, J. Giesen, S. Laue.
Why Capsule Neural Networks Do Not Scale: Challenging the Dynamic Parse-Tree Assumption, AAAI 2023. - J. Giesen, J. Klaus, S. Laue, N. Merk, and K. Wiedom.
Convexity Certificates from Hessians, NeurIPS 2022. - S. Laue, M. Blacher, and J. Giesen.
Optimization for Classical Machine Learning Problems on the GPU, AAAI 2022. - S. Laue, M. Mitterreiter, and J. Giesen.
A Simple and Efficient Tensor Calculus, AAAI 2020. - S. Laue, M. Mitterreiter, and J. Giesen.
GENO - GENeric Optimization for Classical Machine Learning, NeurIPS 2019. - J. Giesen, S. Laue, A. Loehne, and Ch. Schneider.
Using Benson’s Algorithm for Regularization Parameter Tracking, AAAI 2019. - S. Laue, M. Mitterreiter, and J. Giesen.
Computing Higher Order Derivatives for Matrix and Tensor Expressions, NeurIPS 2018. - K. Blechschmidt, J. Giesen, and S. Laue.
Tracking of Approximate Solutions of Parameterized Optimization Problems over Multi-Dimensional (Hyper-)Parameter Domains, ICML 2015. - J. Giesen, S. Laue, and P. Wieschollek.
Robust and Efficient Kernel Hyperparameter Paths with Guarantees, ICML 2014. - J. Giesen, S. Laue, J. Mueller, and S. Swiercy.
Approximating Concavely Parameterized Optimization Problems, NIPS 2012. - S. Laue.
A Hybrid Algorithm for Convex Semidefinite Optimization, ICML 2012.