Paper accepted at IEEE CNS 2024
20 August 2024, by Mathias Fischer
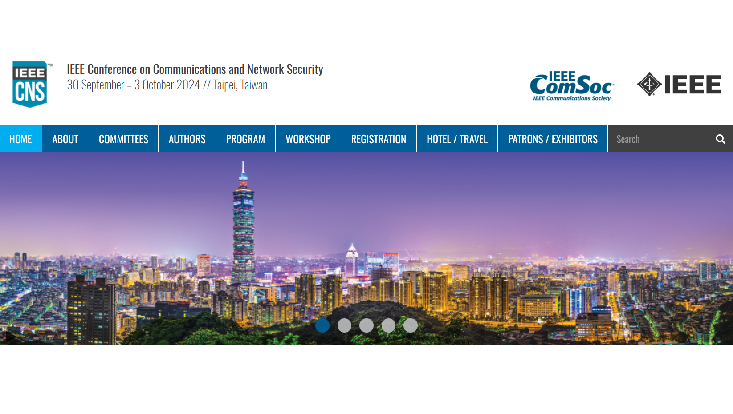
Photo: https://cns2024.ieee-cns.org/
We are happy to announce that our paper, "Privacy-preserving Network Anomaly Detection on Homomorphically Encrypted Data", has been accepted for publication at the twelfth annual IEEE Conference on Communications and Network Security (IEEE CNS) 2024.
The paper addresses the issue of privacy-preserving anomaly detection within Intrusion Detection Systems (IDS) operating in cloud environments, evaluating the applicability of two encryption schemes for secure network traffic anomaly detection.
We look forward to presenting our findings at the conference in Taipei, Taiwan (September 30 - October 3, 2024) and seek an exchange with international researchers.
Paper Abstract:
With the increasing reliance on cloud computing for managed security services, sensitive data, such as network or host data, is increasingly not processed on-premise anymore. To protect the privacy of this sensitive data while processed in the cloud we propose a framework that combines homomorphic encryption (HE) with privacy-preserving machine learning (PPML) techniques, to run Intrusion Detection Systems (IDS) in the cloud, without exposing sensitive data to cloud providers. Our work evaluates the applicability of two HE schemes for network traffic anomaly detection, focusing on computational efficiency and compatibility with PPML. We adopt optimization strategies, namely quantization and feature vector reduction, to enhance computational efficiency and ensure the scalability of anomaly detection tasks in cloud-based environments. Furthermore, we conduct a runtime analysis to evaluate the practical feasibility of integrating HE with PPML for IDS. Our findings indicate that while incorporating HE introduces a computational overhead, there are realistic scenarios where the proposed system can be effectively applied, offering a balance between privacy preservation and operational requirements.