Paper accepted at IFIP SEC'24
16 May 2024, by Mathias Fischer
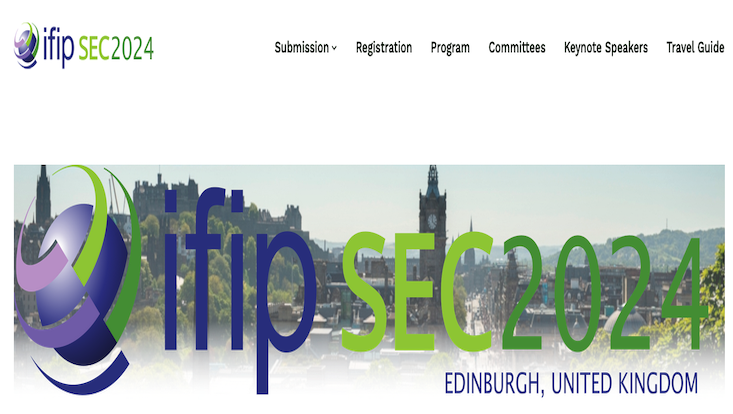
Photo: https://ifipsec2024.co.uk/
Our paper, ”Detecting Web Bots via Keystroke Dynamics” was accepted for publication at the Security and Privacy Protection in Information Processing Systems conference (IFIP SEC 2024).
The paper addresses the escalating issue of sophisticated malicious web bots, proposing a novel detection method using keystroke dynamics modeled by state-of-the-art diffusion models, demonstrating improved performance in classifiers and feasibility in bot detection based solely on keystroke timings.
Paper Abstract:
The increasing presence of malicious web bots within the digital ecosystem has not only escalated operational costs for web services but also significantly deteriorated user satisfaction, especially in sectors like online gaming and social media. These bots engage in a range of unauthorized activities, contributing to a complex threat landscape. The situation is further complicated by rapid advancements in AI, which not only increase the demand for data, often sourced through unauthorized web scraping by bots, but also blur the lines between human and computer interactions. Enhanced image recognition capabilities allow AI to effectively bypass CAPTCHA systems, and the deployment of large language models provides sophisticated reasoning abilities. In response, this paper introduces an approach to mitigate these challenges by leveraging keystroke dynamics as a means for bot detection. Classifiers that differentiate between humans and bots based on keystroke patterns necessitate the use of synthetically generated keystroke data for training purposes. Such data is typically generated using generative AI models; however, it often lacks contextual accuracy, leading to easily distinguishable keystrokes and consequently affecting the classifiers' efficacy. To address this issue, our method models keystroke dynamics in a key-dependent fashion, employing a state-of-the-art diffusion model to produce data of superior quality. We further illustrate that classifiers, particularly those based on Transformer and LSTM architectures, exhibit enhanced performance when retrained with this refined data. We furthermore assess the feasibility of bot detection by exclusively analyzing keystroke timings without including key identity, which we find feasible with only a marginal performance decrease.