Monastery Apple Dataset
Welcome to the Monastery Apple Dataset (MAD) webpage. We introduced this dataset for semi-supervised apple detection in our WACV'24 paper S³AD: Semi-supervised Small Apple Detection in Orchard Environments. We also provide supplementary material, a poster, a video, and code for the paper.
Dataset
To facilitate semi-supervised apple detection, we propose the Monastery Apple Dataset (MAD). The data was acquired in collaboration with a monastery in Bad Oldesloe, Germany. We collected video data from 16 trees of the monastery’s apple orchard with a DJI-Mini 3 Pro drone that has a resolution of 4k (3840 × 2160). The data collection was conducted over the course of one month in September 2022 and during different lighting conditions to ensure diversity. To generate the training, validation, and test splits, we first assigned the videos of 6 (training), 2 (validation), and 4 (test) trees to the respective splits. From the videos, we manually selected images for annotation to maximize data diversity. The training, validation, and test splits consist of 66, 12, and 27 frames with 10,089, 1,288, and 3,290 annotated instances as shown in the following table.
Split | Images | Labeled Instances |
train | 66 | 10,089 |
val | 12 | 1,288 |
test | 27 | 3,290 |
unlabeled | 4,440 | n/a |
The apples were manually annotated with bounding boxes. An example image with ground truth from our dataset is shown below. Moreover, we automatically assigned three properties to each annotated apple representing the relative size, the level of occlusion, and the lighting conditions (see below). This enables us, to evaluate the influence of such conditions on our system. The 4,440 unlabeled images in the training split are composed of frames from the 6 training trees that were not annotated and frames from the four remaining videos/trees that were not assigned to the other splits.
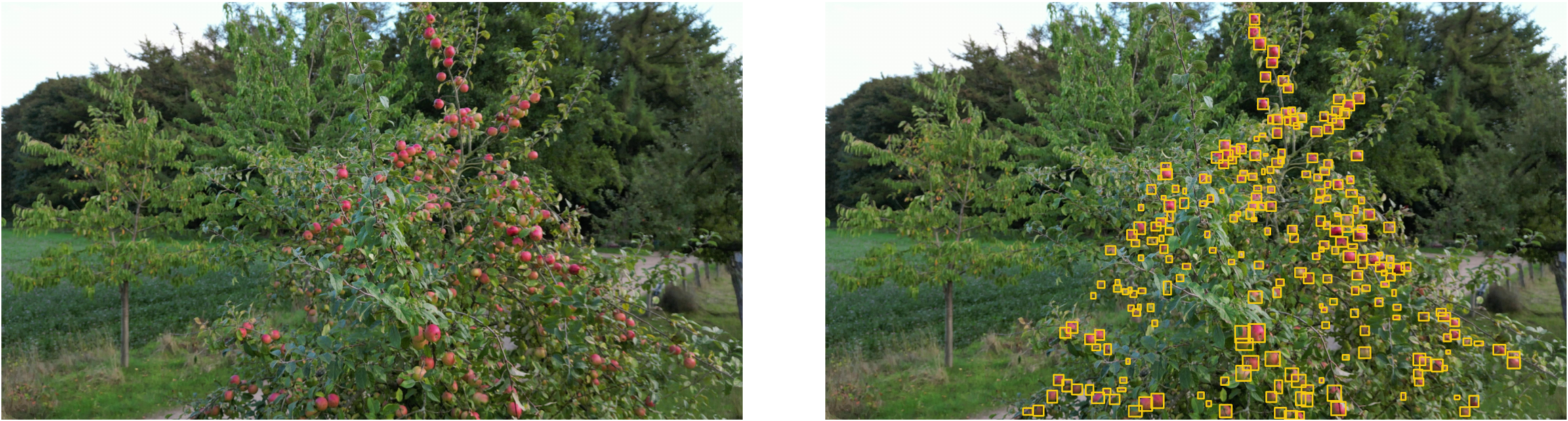
Download, Data Usage, and License
You can download the dataset MAD here.
The data is stored in a zip file containing five folders. For the training, validation, test, and unlabeled splits (used in training), there exists one folder each with the respective images (jpg
s). The annotations are collected in COCO-style in three annotation files (instances_<SPLIT>.json
) in the annotations
folder.
The dataset including images and annotations are provided under the CC BY-NC-SA license.
If you have any questions or comments, email Christian Wilms(christian.wilms"AT"uni-hamburg.de).
How to Cite
If you use this dataset, please cite our paper:
@inproceedings{JohansonEtAlWACV2024,
title = {{S³AD}: Semi-supervised Small Apple Detection in Orchard Environments},
author={Johanson, Robert and Wilms, Christian and Johannsen, Ole and Frintrop, Simone},
booktitle = {Winter Conference on Applications of Computer Vision (WACV)},
year = {2024}
}
Acknowledgments
We express our gratitude to Jan-Gerrit Habekost, Silas Ueberschaer, and Jan Willruth for their support in this project. We also thank Kloster Nütschau for allowing us to capture the drone footage of their apple orchard.