Accepted Papers at LREC 2022
12 July 2022
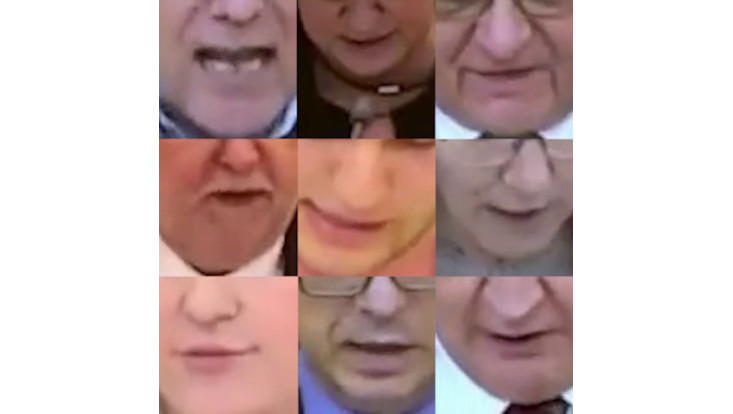
Photo: Hessian Parliament Public Data
Knowledge Technology has got two papers accepted at the 13th Language Resources and Evaluation Conference (LREC 2022). The Conference has been held on June 20-25, 2022 at the Palais du Pharo, Marseille, France.
First Paper
Title: A Multimodal German Dataset for Automatic Lip Reading Systems and Transfer Learning
Authors: Gerald Schwiebert, Cornelius Weber, Leyuan Qu, Henrique Siqueira, Stefan Wermter
Abstract:
Large datasets as required for deep learning of lip reading do not exist in many languages. In this paper we present the dataset GLips (German Lips) consisting of 250,000 publicly available videos of the faces of speakers of the Hessian Parliament, which was processed for word-level lip reading using an automatic pipeline. The format is similar to that of the English language LRW (Lip Reading in the Wild) dataset, with each video encoding one word of interest in a context of 1.16 seconds duration, which yields compatibility for studying transfer learning between both datasets. By training a deep neural network, we investigate whether lip reading has language-independent features, so that datasets of different languages can be used to improve lip reading models. We demonstrate learning from scratch and show that transfer learning from LRW to GLips and vice versa improves learning speed and performance, in particular for the validation set.
Links: The GLips dataset has been published here.
Second Paper
Title: Conversational Analysis of Daily Dialog Data using Polite Emotional Dialogue Acts
Authors: Chandrakant Bothe, Stefan Wermter
Abstract:
Many socio-linguistic cues are used in conversational analysis, such as emotion, sentiment, and dialogue acts. One of the fundamental cues is politeness, which linguistically possesses properties such as social manners useful in conversational analysis. This article presents findings of polite emotional dialogue act associations, where we can correlate the relationships between the socio-linguistic cues. We confirm our hypothesis that the utterances with the emotion classes Anger and Disgust are more likely to be impolite. At the same time, Happiness and Sadness are more likely to be polite. A less expectable phenomenon occurs with dialogue acts Inform and Commissive which contain more polite utterances than Question and Directive. Finally, we conclude on the future work of these findings to extend the learning of social behaviours using politeness.
Links: Code of this work has been open-sourced here.