New Paper Published at IEEE-RAL
2 December 2024
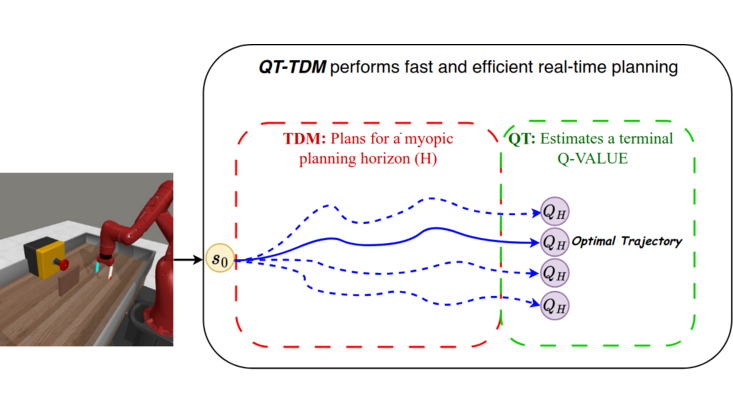
Photo: UHH Knowledge Technology
Our group has a new paper published in IEEE Robotics and Automation Letters (RA-L). Here's more information about the paper:
Title: QT-TDM: Planning With Transformer Dynamics Model and Autoregressive Q-Learning
Authors: Mostafa Kotb, Cornelius Weber, Muhammad Burhan Hafez, Stefan Wermter
In this work, we developed a new model-based algorithm, QT-TDM, which overcomes the slow and computationally inefficient inference associated with Transformer Dynamics Models (TDMs).
QT-TDM consists of two modules:
a) Transformer Dynamics Models (TDM).
b) Q-Transformer (QT).
Both modules are trained separately, but they cooperate during inference by performing Model Predictive Control with a myopic planning horizon and a terminal value function. The terminal Q-value is estimated by the model-free Q-Transformer model. Experiments show that QT-TDM is superior in performance and sample efficiency compared to existing Transformer-based and Recurrent-based reinforcement learning (RL) models while achieving fast and computationally efficient inference.