Dr. Xavier Hinaut
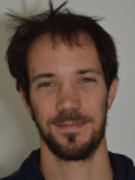
Photo: UHH/Knowledge Technology
Research Associate
Knowledge Technology
Address
Office
Contact
Webpage: | www.xavierhinaut.com |
Short Curriculum Vitae
Jan 2013 | PhD in Computer Science, Cortical Networks for Cognitive Interaction team, INSERM - Stem Cell and Brain Research Institute, University of Lyon I, France |
Since 2015 | Marie Curie Fellow, Knowledge Technology Research Group, Department of Computer Science, University of Hamburg, Germany |
NEW! IJCAI 2015 video:
"Humanoidly Speaking": How the Nao humanoid robot can learn the name of objects and interact with them through common speech.
IJCAI 2015 video competition link.
Software: Take a look at "Syntactic Reservoir Model" and "DOCKS" on the sofware webpage to download and try the source code to make the same experiment.
Several research topics for bachelor/master students available: Look for "How children learn languages? Recurrent Neural Networks to enable robots to learn (any) language!" at the Research Topics for Students webpage or look at this pdf for more details.
Marie Curie IEF project
"EchoRob": Echo State Networks for Developing Language Robots
Project webpage
In this project, we propose to build a biologically plausible model of sentence comprehension based on recurrent neural networks called reservoirs. Human sentence comprehension is mainly handled by prefrontal cortex areas, which have highly recurrent connectivity. In both biological and artificial neural networks this recurrence is supposed to enable the management of different aspects of time such as working memory and contextual information processing.
Here, we propose to develop the Reservoir Computing (RC) paradigm — in particular Echo State Networks (ESN) with incremental learning — to model language comprehension at the sentence level given sequential inputs of words or phonemes. Based on our initial research, a model processing syntactic sentence structures was able to demonstrate generalisation and online prediction capabilities while processing sequential input. For less frequent inputs, the model provided potential explanation for human electrophysiological data. Building on this research, a new model is proposed with the following objectives:
- processing of all semantic information enabling contextual processing and richer representation of meaning,
- implementing incremental learning with noisy supervision enabling realistic developmental language acquisition from simple to complex sentences,
- demonstrating that the model can learn from naïve user's utterances in several languages, and
- demonstrating the ability of this model when embodied in a robot to acquire extended language capabilities through human-robot interaction accounting for developmental schemes.
See more on my personal webpage: www.xavierhinaut.com