Non-linear Spatial Filtering
The focus of this research area are (DNN-based) joint spatial and tempo-spectral non-linear filters for multi-channel speech enhancement and separation.
For a long time, the dominant approach to multi-channel speech enhancement has been to employ a beamformer for linear spatial filtering and a separate tempo-spectral (non-linear) post-filter for post-processing. Inspired by the rise of deep learning techniques in the last ten years, many researchers are currently proposing new end-to-end DNN-based systems, which map the noisy multi-channel recordings to an enhanced single-channel output signal. In this way, a DNN implements a filter that is not only non-linear but also jointly performs the spatial and tempo-spectral filtering.
In our research, we aim to gain a better understanding of such joint spatial and tempo-spectral non-linear filters with respect to their theoretical properties as well as practical implementation with DNNs. Our latest research efforts target the following topics:
- Investigating the performance potential of a joint non-linear filter in comparison with the beamformer plus post-filter model from a statistical perspective (based on analytical estimators).
- Design and analysis of a DNN-based joint spatial and tempo-spectral filter. Our focus is on the interaction between between spatial, spectral and temporal information and how these can be best exploited by a neural network architecture.
- Development of a steerable filter with controllable look direction for speech separation.
A good theoretical understanding helps us to build high-performing systems that can be used in practise. Please take a look at the video of our real-time demo system.
Statistical perspective on the performance potential of non-linear spatial filters
While single-channel speech enhancement approaches (traditional statistics-based or based on a deep neural network (DNN)) make use of tempo-spectral signal characteristics to perform the enhancement, multichannel approaches can additionally leverage spatial information contained in the noisy recordings obtained using multiple microphones. Today, the majority of new devices is equipped with multiple microphones, which emphasizes the practical relevance of spatial information processing. Traditionally, spatial filtering is achieved by so-called beamformers that aim at suppressing signal components from other than the target direction. The most prominent example is the filter-and-sum beamforming approach, which achieves spatial selectivity by filtering the individual microphone signals and adding them. This results in a linear operation with respect to the noisy input. The filter weights are designed to optimize some performance measure. For example, minimizing the noise variance subject to a distortionless constraint leads to the well-known minimum variance distortionless response (MVDR) beamformer. The output of the linear beamformer is then further processed by an independent single-channel tempo-spectral post-filter.
While it is convenient to independently develop the spatial and spectral processing stage, the theoretical analyses consolidated in our paper [1] show that this is optimal in the minimum mean-squared error (MMSE) sense only under the restrictive assumption that the noise signal is following a Gaussian distribution. In our work [1-3], we demonstrate based on evaluations that a joint spatial and spectral non-linear filter indeed enables considerable performance improvements over the composed setup in non-Gaussian noise sencarios revealing the theoretical results to be highly relevant for practical applications.
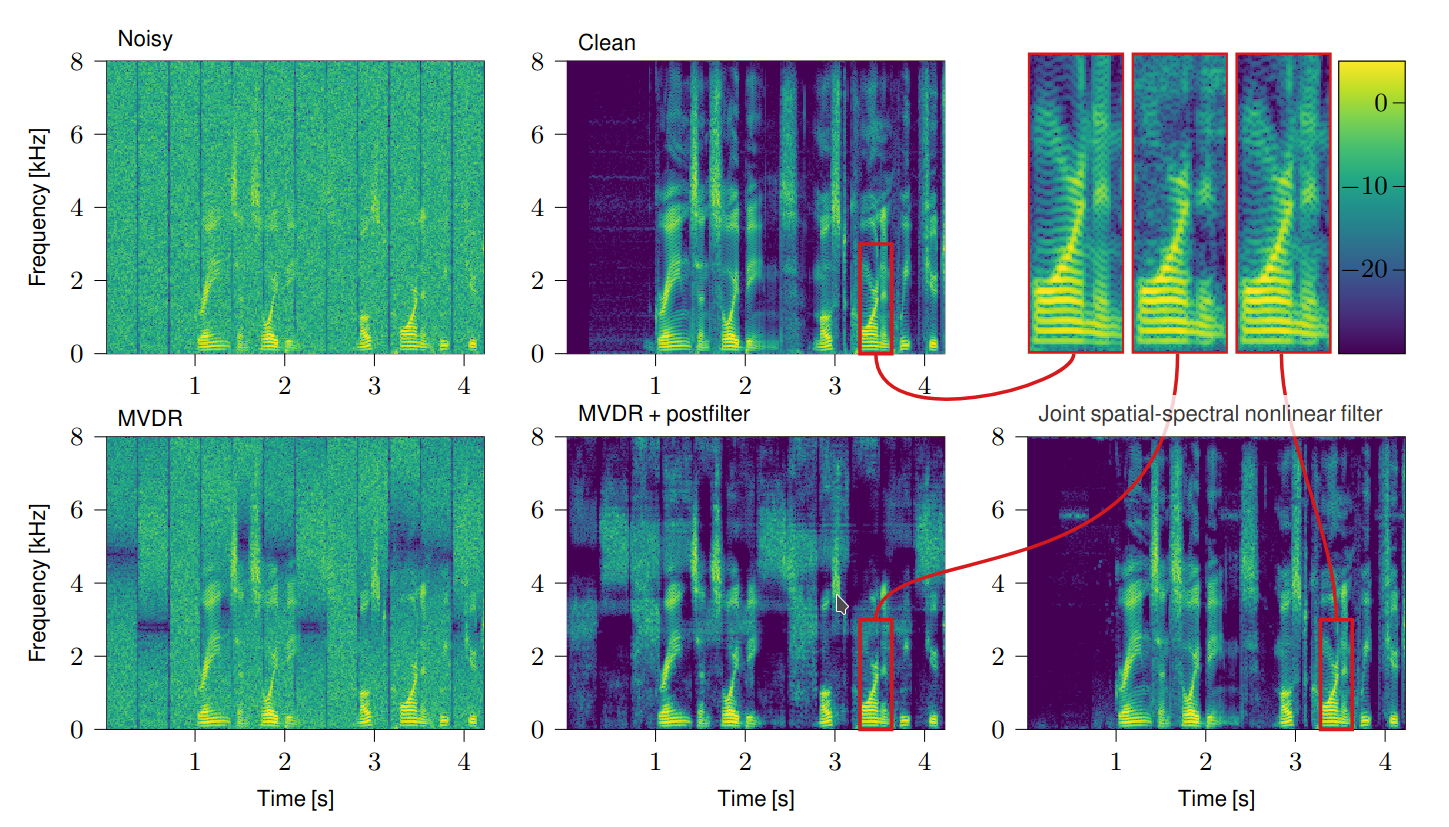
Figure 2 depicts experiment results published in [1] for an inhomogeneous noise field created by five interfering noise sources. The noise and target signals are captured by a two-channel microphone array. As the number of interfering sources is larger than the number of microphones, the enhancement capabilities of traditional two-step approaches combining a linear spatial filter and a postfilter are limited. In the presented example, the interfering sources are emitting Gaussian bursts with only one interfering source being active per time segment. The uniform green coloration of the vertical stripes in the noisy spectrogram reflects the spectral stationarity. The vertical dark blue lines separate segments with different spatial properties. We would like to emphasize the visible and audible difference between the result obtained by an MVDR combined with a postfilter (middle in the bottom row) and the analytical joint spatial-spectral non-linear filter (last in the bottom row). While the latter can recover the original clean signal almost perfectly, the MVDR plus postfilter suffers from audible speech degradation and residual noise. The audio examples can be found here. We conclude from the experiment that a non-linear spatial filter that combines the spatial and spectral processing stage is capable of eliminiating more than the traditional M-1 sources from M microphones without spatial adaptation and, thus, has some notably increased spatial selectivity.
In our work [1-3], we evaluated the performance of the non-linear spatial filter in different non-Gaussian noise scenarios. We find a notable performance improvement also in other experiments with heavy-tailed noise distributions, interfering human speakers, and real-world noise recordings from the CHiME3 database.
Do not miss the exciting audio examples for these experiments here.
Investigation of DNN-based joint non-linear filters
- Is non-linear as opposed to linear spatial filtering the main factor for good performance?
- Or is it rather the interdependency between spatial and tempo-spectral processing?
- And do temporal and spectral information have the same impact on spatial filtering performance?
- FT-JNF: joint spatial and tempo-spectral non-linear filter (all three sources of information)
- F-JNF: spatial-spectral non-linear filter
- T-JNF: spatial-temporal non-linear filter
- FT-NSF: non-linear spatial filter (fine-grained tempo-spectral information is excluded, global tempo-spectral information is accessible)
- F-NSF: non-linear spatial filter (fine-grained spectral information is excluded, global spectral information is accessible)
- T-NSF: non-linear spatial filter (fine-grained temporal information is excluded, global temporal information is accessible)
- LSF: oracle MVDR baseline
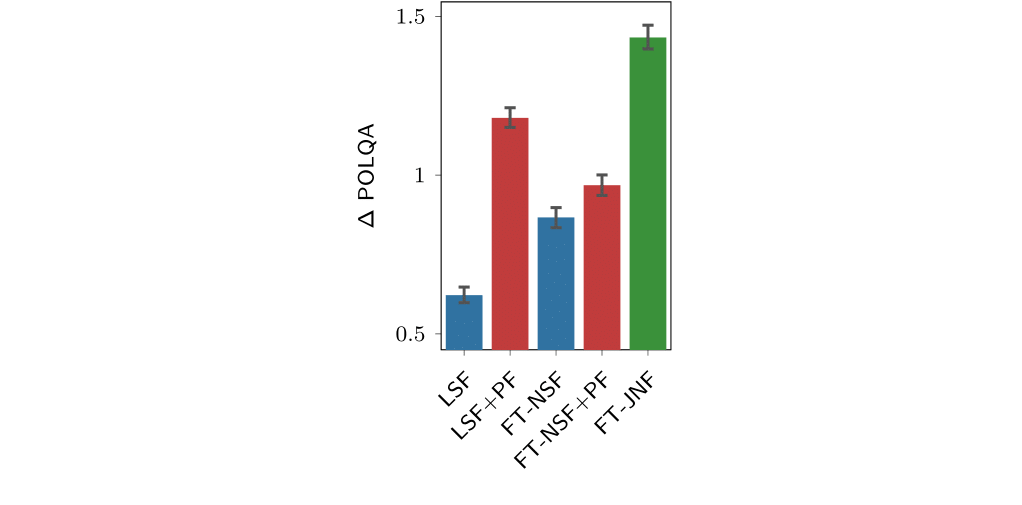
The results displayed in Figure 3 show that the non-linear spatial filter (FT-NSF) outperforms the oracle linear spatial filter (LSF, which is an oracle MVDR beamformer). Its seems that the more powerful processing of spatial information leads to a clear performance advantage. However, the picture changes when post-filtering is included. While a independent tempo-spectral post-filter leads to good results when applied to the output of a linear and distortionless MVDR beamformer, the non-linear spatial filter does not benefit that much. We can explain this by the fact that the non-linear spatial filter introduced quite some speech distortions that cannot be fixed by a mask-based post-filter. In contrast, if spatial and tempo-spectral information are processed jointly, the overall best performance is obtained. These results highlight the importance of interdependencies between spatial and tempo-spectral information.
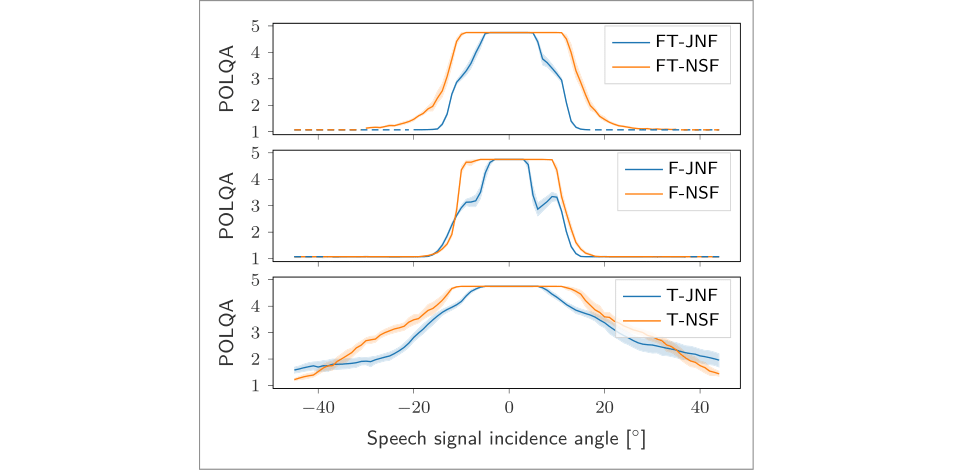
In [5], we have investigated the spatial selectivity of the different filters. A visualization of spatial selectivity of the learnt filters are shown in Figure 4. We find that that joint processing in particular of spatial and spectral information increases the spatial selectivity.
Please find audio examples here.
Steerable deep non-linear filters for speaker extraction and separation
In the publications [6,7], we introduce a steering mechanism for the DNN-based non-linear spatial filters. By conditioning the neural network on the direction of arrival (DOA) of the target speaker, we can extract any speaker from the mixture or all of them if their DOA is known. The conditioning mechanism is simple but effective:
- We represent the target speaker's DOA with a one-hot encoded vector with 180 entries. This corresponds to a two-degree resolution.
- The one-hot encoded vector is feed through a linear.
- The output of the linear layer is used as an initial state for the first LSTM layer in our network which processes spatial and spectral information.
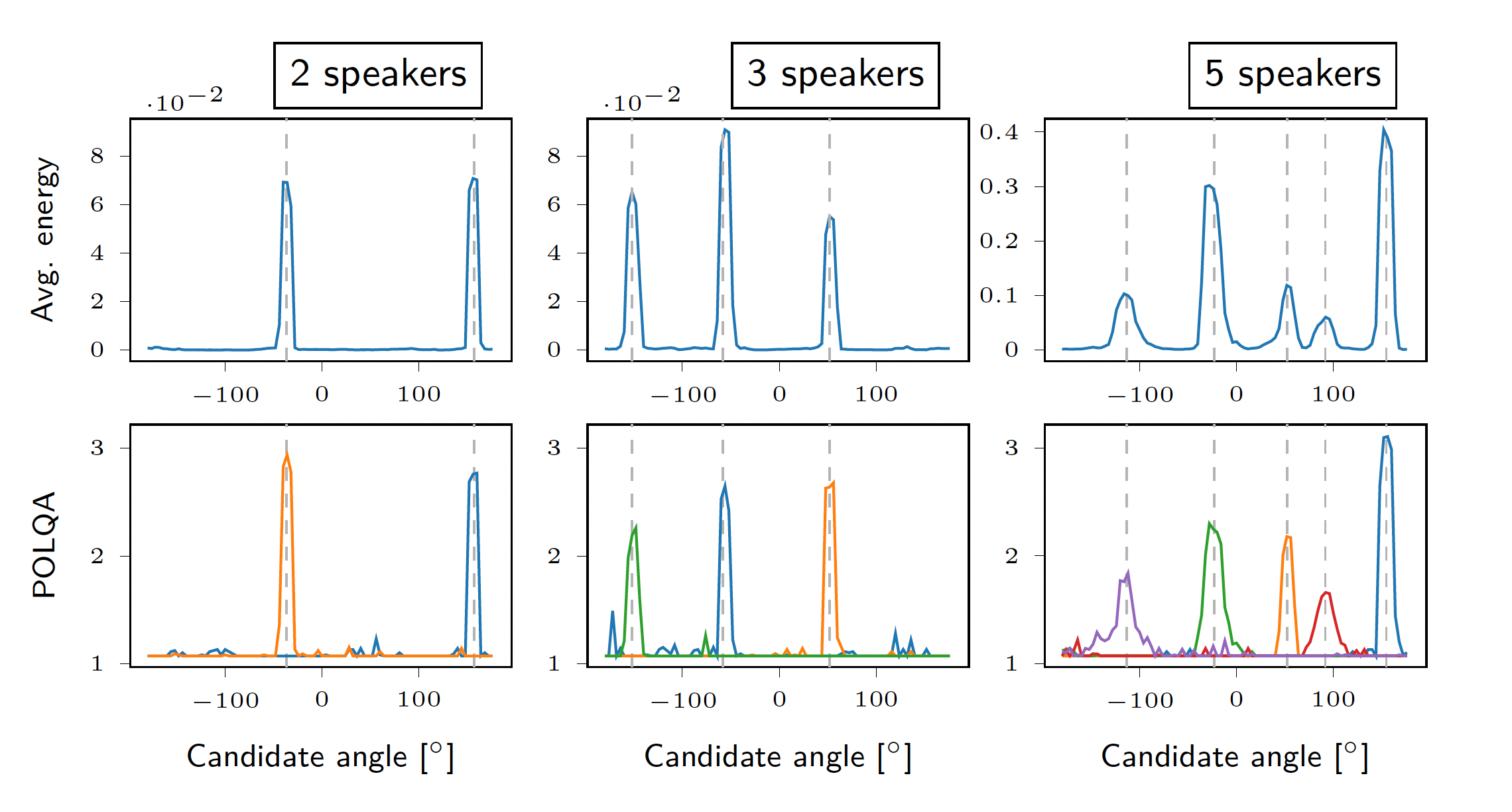
Figure 5 shows results for mixtures of two, three and five speakers. The speaker DOAs are shown by the dashed gray lines. The DNN-based filter is then steered in all possible angles. The top row shows the energy of the resulting signal and the bottom row the POLQA scores using each speaker as reference. We observe distinct peaks around the DOAs of the different speakers, which shows high spatial selectivity of the resulting filter.
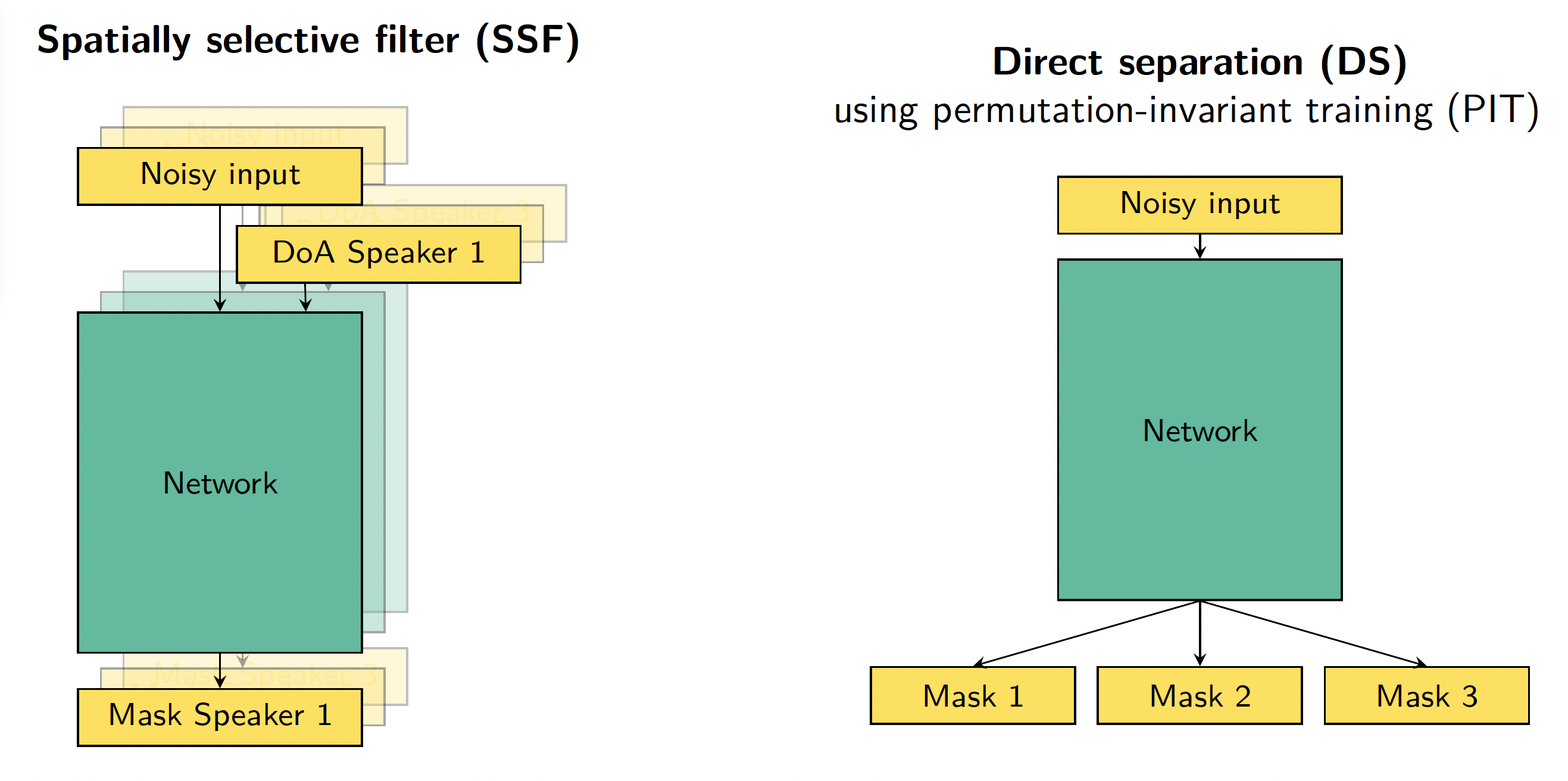
We can use our spatially selective filter (SSF) not only to perform speaker extraction but also speech separation. Here we require the DOAs of the different speakers and can then steer the filter to extract the individual speakers. A visualization of this approach is shown in the left plot of Figure 6. In contrast, a more common approach, which we call direct sepration (DS), is to feed the noisy mixture to the network and train it to estimate an output for each of the speakers in the mixture. Clearly, this requires knowledge of the number of speakers in advance. A visualization of this DS approach using permutation-invariant training (PIT) is shown on the right side of Figure 6.
It is reasonable to assume that the best performance on a difficult speech separation task with a high number of speakers will be achieved by a filter that has a high spatial selectivity. The training strategy of the SSF explicitly focuses on the spatial selectivity of the filter, while the DS approach requires to learn this implicitly from training examples. In Figure 7, we compare the performance of both approaches for two network architectures of different sizes. The results are taken from [7].
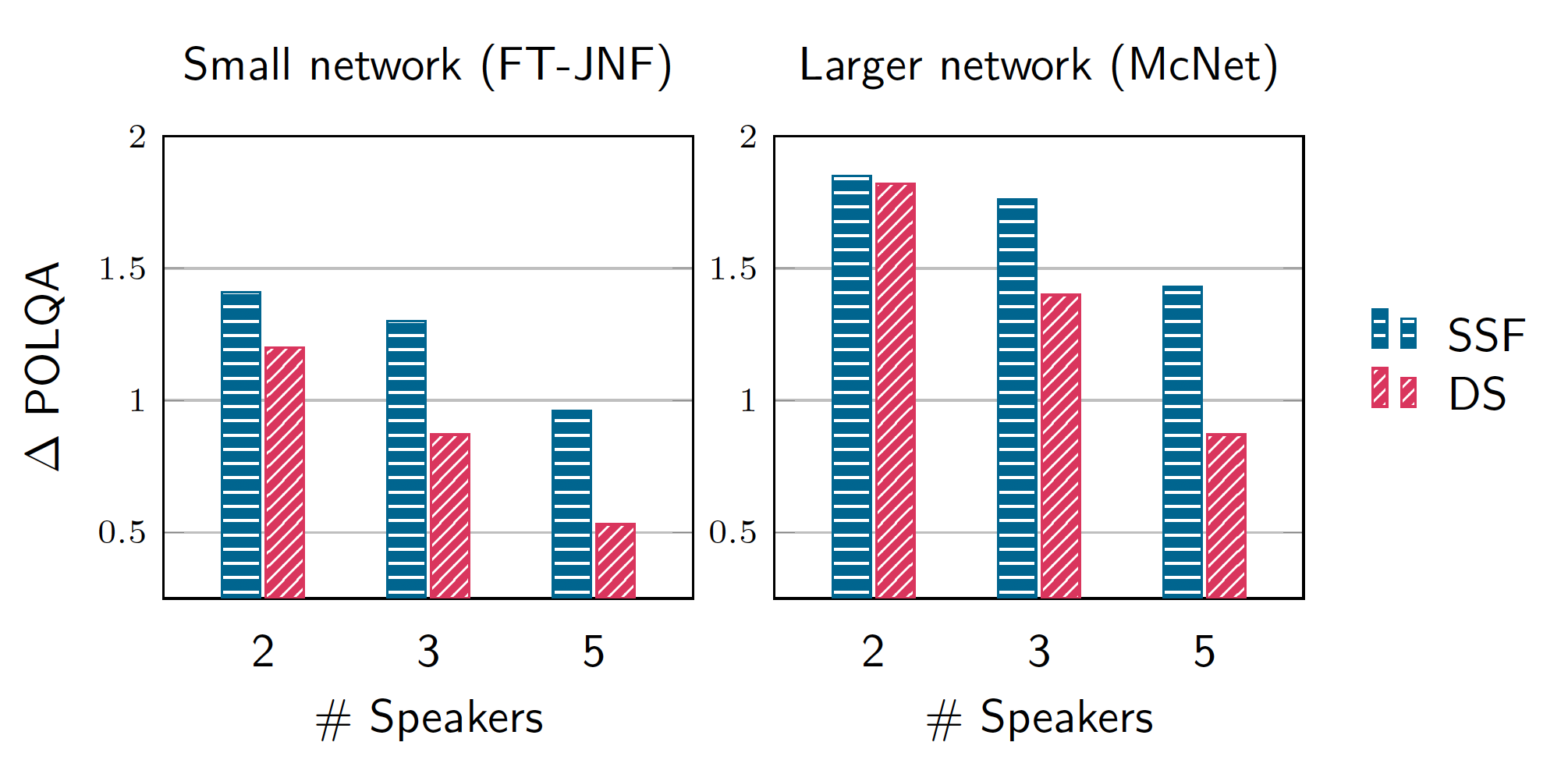
From the results in Figure 7, it can be seen that the SSF approach outperforms the DS approach trained with PIT in particular for a smaller network size or a more difficult scenario with a higher number of speakers in the mixture. In our publication [7], we also find that the SSF approach does not only deliver better performance than DS but also enables better generalization to unseen scenarios, e.g., close speakers or additional music noise sources. Some of these situations are also demonstrated in the video at the top of this page.
Publications
- Kristina Tesch, Robert Rehr, Timo Gerkmann. "On Nonlinear Spatial Filtering in Multichannel Speech Enhancement", Interspeech, Graz, Austria, Sep. 2019. [pdf]
- Kristina Tesch, Timo Gerkmann, "Nonlinear Spatial Filtering For Multichannel Speech Enhancement in Inhomogeneous Noise Fields", IEEE Int. Conf. Acoust., Speech, Signal Process. (ICASSP), Barcelona, Spain, May 2020.[doi]
- Kristina Tesch, Timo Gerkmann, "Nonlinear Spatial Filtering in Multichannel Speech Enhancement", IEEE/ACM Trans. Audio, Speech, Language Proc., 2021. [doi] [arxiv] [audio]
- Kristina Tesch, Nils-Hendrik Mohrmann, Timo Gerkmann, "On the Role of Spatial, Spectral, and Temporal Processing for DNN-based Non-linear Multi-channel Speech Enhancement", Interspeech, Incheon, South Korea, 2022. [arxiv][audio]
- Kristina Tesch, Timo Gerkmann, "Insights into Deep Non-linear Filters for Improved Multi-channel Speech Enhancement", IEEE/ACM Trans. Audio, Speech, Language Proc., 2023. [arxiv][audio]
- Kristina Tesch, Timo Gerkmann, "Spatially Selective Deep Non-linear Filters for Speaker Extraction", IEEE Int. Conf. Acoust., Speech, Signal Process. (ICASSP), Rhodes Island, Greece, June 2023. [arxiv][audio]
- Kristina Tesch, Timo Gerkmann, "Multi-channel Speech Separation Using Spatially Selective Deep Non-linear Filters", IEEE/ACM Trans. Audio, Speech, Language Proc., 2024 [doi][arxiv][audio]