Kolloquium SoSe 2024
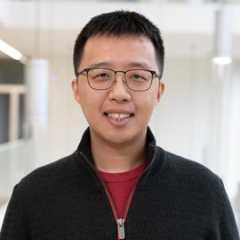
Dr. Xiao Zhang
CISPA Helmholtz Center for Information Security
Wann: Mo, 17.06.2024, 16:15 Uhr
Wo: Konrad-Zuse-Hörsaal (Raum B-201)
Thema
Uncovering the Limits of Adversarial Machine Learning
Sprache: English
Abstract
It has been repeatedly shown that modern machine learning models are highly vulnerable to adversarial manipulations. Numerous efforts have been made to design attack methods to realize the attacker’s goal and develop defense mechanisms to improve model robustness. However, due to the variations in threat models, learning algorithms and data distributions, there is a lack of systematic and principled approaches to reasoning what causes the adversarial vulnerability of machine learning models. In the first part of this talk, I will introducea concentration estimation framework designed to measure the intrinsic robustness limits against adversarial perturbations and discuss its implications for understanding the fundamental causes of adversarial vulnerability behind state-of-the-art robustly trained models. In the second part of this talk, I will introduce our recent work on characterizing the optimal indiscriminate data poisoning attacks against linear learners, and then discuss the key task-relevant properties that help explain the drastic difference in poisoning attack effectiveness across datasets. Finally, I will briefly talk about our ongoing work of leveraging the learning dynamics of stochastic gradient descent with neural networks for more accurate characterization of optimal membership inference and conclude with future research directions I would like to pursue.
Bio
Xiao Zhang is a tenure-track faculty member at CISPA Helmholtz Center for Information Security in Saarbrücken, Germany. He obtained his PhD in computer science in 2022 from the University of Virginia in Charlottesville, USA, where he worked with Prof. David Evans. His research interests lie in characterizing the fundamental limits of adversarial machine learning using principled approaches from learning theory, optimization and statistics, then leveraging the theoretical insights to build better robust machine learning systems. He also serves as a member of the European Laboratory for Learning and Intelligent Systems (ELLIS).